Data Science in Retail
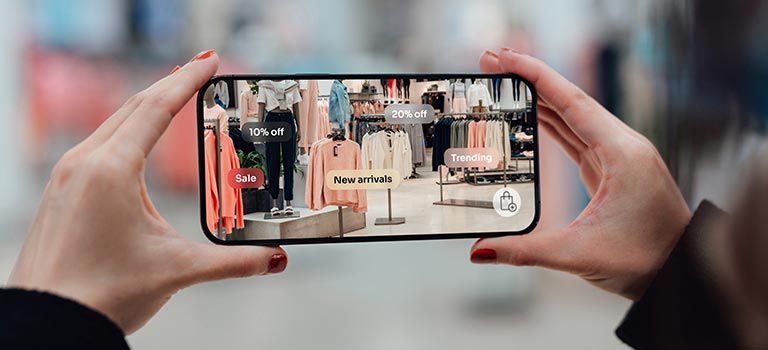
Opportunities in Retail Data Science
The Promise of Big Data
Retailers can turn insights from big data into profitable margins by developing insight-driven plans, investing in big data talent, and investing in existing employees.
This has created a need for a new brand of analyst – the data scientist. His or her job is to burrow into the mountain of big data (internal or external, structured or unstructured) to find gold. In other words, the actions retailers can take to reduce costs and increase sales.
Sponsored Schools
SPONSORED
Customer Experience
- Personalized recommendations and multi-level reward programs based on purchase preferences, online data, smartphone apps, etc.
- Sentiment analysis of social media streams, call center records, product reviews, etc. for customer feedback and market insights
- Predictive analytics for the enhancement of the customer experience across all channels and devices, online and off
Merchandising
- Improved layouts, promotional displays and product placements using heat sensors and image analysis to identify behavioral patterns
- Identification of shopping trends and cross-selling opportunities through video data analysis
- Higher daily profits through a combination of internal and external data (e.g. economic forecasts, weather and traffic reports, holiday and seasonal trends)
- Faster revenue growth through detailed market basket analysis
- Insights using product sensors that relay real-time information on post-purchase use
Marketing
- Location-based and personalized offers on mobile devices
- Real-time pricing using “second by second” metrics (e.g. supply chain and inventory data, competitor pricing, market and consumer behavior data)
- Targeted campaigns using analytics to segment consumers, identify the most appropriate channels and achieve optimal ROI
- Tailored offers through online behavioral analysis and web analytics
Supply Chain Logistics
- Improved, real-time inventory tracking and management
- Route optimization and more efficient transportation using GPS-enabled big data telematics
- Demand-driven forecasting through a combination of structured and unstructured data
- More effective supplier negotiations based on in-store records
The Omni-Experience
From the moment the product leaves the manufacturer, to its journey to the warehouse or store floor, to its purchase and appreciation, the retailer is typically looking for maximum efficiency in every department.
In a way, we’re going back to the old model of the general storekeeper. The storekeeper knew the story of every person in town. They knew what vegetables you liked to eat, what clothes you preferred to wear, how much you could afford to spend that week, your family predisposition to halitosis… The storekeeper used it to make recommendations that fit you and no other. That’s the relationship retailers are aiming for.
Enter the Vendors
Retailers looking for assistance will find a host of vendors at their disposal. In addition to creating their own data science teams, companies are partnering with big names like IBM and Oracle and/or installing software suites like SAS that can provide them with advanced analytics, business intelligence and data management.
Of these names, IBM is one of the most prominent in the retail field. It spent the first decade of the 21st century snapping up companies like Unica and partnering with players like Teradata, an enterprise analytics software company, and BloomReach (which uses predictive analytics to show customers more relevant organic search content).
Data Risks & Regulations
The Perils of Using Consumer Data
The Federal Trade Commission (FTC) is primarily responsible for regulating e-commerce activities. They oversee everything from commercial emails to online advertising, and see to it that consumer privacy is respected.
And privacy is where the law and big data collide. Thanks to a slew of multi-platform marketing efforts – loyalty programs, online transactions, mobile and social media campaigns – retailers now store and handle an enormous amount of consumer data, including credit card and social security numbers. Much of this information is moving off servers and onto the cloud.
This leaves retailers vulnerable. In 2019, Verizon’s 2020 Data Breach Investigations Report found that data breaches could be attributed to the retail industry in both combined online and point of sale fronts.
Equal Opportunities for Equal Credit
Then there’s the law. As FTC Chair Edith Ramirez points out, the use of big data algorithms must fall within the Equal Credit Opportunity Act (ECOA). Established in 1974, the ECOA makes it unlawful for any creditor to discriminate against any applicant with respect to any aspect of a credit transaction:
- On the basis of race, color, religion, national origin, sex or marital status, or age (provided the applicant has the capacity to contract);
- Because all or part of the applicant’s income derives from any public assistance program; or
- Because the applicant has in good faith exercised any right under the Consumer Credit Protection Act.
Retailers must be careful that their predictive models aren’t automatically making credit decisions that could prove discriminatory.
If the SOX fits…
What’s more, there are very strict regulations about how retailers must handle corporate data. In the wake of the Enron scandal, IT departments at all public companies were handed a new set of rules. Under the Sarbanes-Oxley Act (SOX), businesses were responsible for instituting best practices and controls relating to the:
- Destruction, alteration or falsification of records
- Retention period for the storage of records
- Types of business records that need to be stored, including electronic communications
In addition to keeping their consumer data safe, IT experts have the unenviable task of managing an archive with all their corporate electronic records.
The Danger of Predictive Analytics
But the biggest threat to consumer privacy may come from retailers themselves. As the scope of data science expands, more and more analysts are using predictive models to anticipate customer needs. Retailers want to be telling you what you want before you’re even aware of it.
It’s already begun. In a famous tale told round the world, an angry father stormed into a Target store clutching a slew of baby clothes and crib coupons.
“My daughter got this in the mail!” he blasted. “She’s still in high school, and you’re sending her coupons for baby clothes and cribs? Are you trying to encourage her to get pregnant?”
They weren’t, of course. What Target’s algorithms were doing, however, were tracking his daughter’s purchase history. When she started stocking up on unscented lotion and loading up on vitamin supplements, the store was able to predict her pregnancy. They could even pinpoint her due date.
These kinds of tactics, unsurprisingly, creep customers out. Target altered their marketing technique to appear less targeted – by mixing in coupons with unrelated items – but gained a lot of bad publicity in the process. It’s a warning that retail data scientists should take seriously.
History of Data Analysis and Retail
“Leave no stone unturned to help your clients realize maximum profits from their investment.” – Arthur C. Nielsen, Sr.
The retail industry has been amassing marketing data for decades. As early as 1923, Arthur C. Nielsen, Sr. created a company solely dedicated to marketing research and buying behavior. Now known as ACNielsen, it was one of the first to the frontier of consumer data analytics.
The gold rush was on:
- In the 1970s, retailers began to use barcodes to scan products at POS (point of sale).
- A decade later, data centers were flooded with information from RFID (radio-frequency identification) tags and surveillance video footage.
Well before the advent of the Internet, savvy retailers were used to slicing and dicing data points.
Toothpaste On Demand
In the late 1980s and early 1990s, Wal-Mart began to take a serious statistical look at its internal supply chain data, including the ins and outs of suppliers’ processes and systems. The goal? To lower costs related to excess inventory.
It worked. Wal-Mart was able to slash its expenses by better management. Wal-Mart also reached out to manufacturing partners like Procter & Gamble (P&G). Together they built a software system that connected P&G to Wal-Mart’s distribution centers.
Whenever P&G products ran low, an automatic alert was sent to the manufacturer. P&G could also monitor the flow of its products through Wal-Mart’s register scanners. Products were shipped as soon as they were needed. Invoicing and payments happened automatically.
Beer and Diapers
Here’s the myth. In the early 1990s, Osco Drug used data mining techniques to discover that men shopping on Thursdays and Saturdays needed two things: diapers for their kids and beer for the weekend. By moving the diapers next to the beer, Osco could sell a lot more of both.
Here’s the reality. In 1992, NCR (the forerunner of Teradata) conducted an analysis for Osco. They looked at 25 stores, peeked into 1.2 million market baskets and pegged over 20 different product pairs – including beer and diapers.
But then Osco did something unusual. It took the data from the NCR study and pinpointed 5,000 sluggish SKUs that were sitting on shelves and collecting dust. When it removed these SKUs, sales of the other items increased. Customers could find what they wanted more quickly.
A Flood of Data
Then came the flood. Retailers who were used to dealing with data on floor layouts were now hit with a tidal wave of information on consumers. Suddenly, contenders like Amazon, eBay, Zappos and Netflix were using data to challenge conventional marketing tactics. It was, as they say, the start of a brave new world.
Incorporated in 1994, Amazon sold its first book in 1995 – Douglas Hofstadter’s Fluid Concepts and Creative Analogies: Computer Models of the Fundamental Mechanisms of Thought. By the fourth quarter of 2001, it had survived the burst of the dot.com bubble and turned its first profit. By the mid-2000s, it was using sophisticated algorithms to recommend items based on customers’ buying patterns.
Data analysts were in heaven. Companies such as Unica (1992) began to realize that data mining and predictive analytics could give businesses a huge competitive advantage. They homed in on e-commerce, showing companies how to maximize their marketing tactics. In 2010, Unica became part of IBM’s Enterprise Marketing Management (EMM) group.
Last updated: June 2020